IFOY FINALIST FOCUS: Pallet Classification System from SICK
10th June 2022
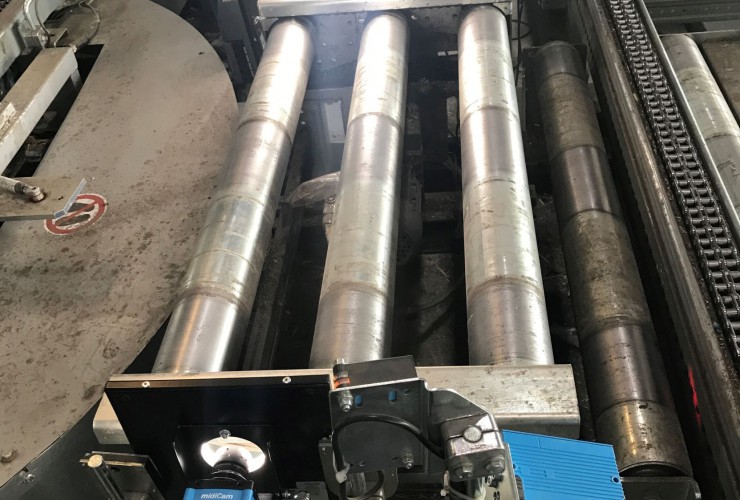
The next in our look at all of the IFOY Award nominated finalists ahead of the winners announcement on 30th June at BMW World is a focus on the PACS (Pallet Classification System) from SICK.
IFOY Category: Special of the Year
Description
SICK’s deep learning-based pallet identification system PACS is used for automated recognition of pallet types. The automated recognition simplifies the process of automatically tagging different pallet types and can be easily integrated in many locations due to its compact design and small footprint.
The hardware of the system consists of two colour cameras for image acquisition, a light barrier array for triggering, and a controller for processing the data and executing the trained neural network. Optionally, other SICK sensors (e.g. barcode scanners) can be integrated to realize additional tasks.
The software tools SICK Appspace and dStudio enable image recording, training, classification and execution of the trained network even without in-depth knowledge of programming or machine learning. Optionally, further SICK sensors can be integrated, which can take over additional tasks..
Innovation
Unlike conventional image processing solutions, the use of deep learning technology in the SICK solution does not require detailed programming knowledge, as the system learns from concrete examples. This has enabled SICK to make pallet identification comparatively simple for the customer. Where the use of trained neural networks would normally require in-depth machine learning knowledge, SICK developed dStudio, a training software that includes a guided process flow. This has made it possible to reduce the large number of setting options of conventional solutions to a minimum. This simplification allows users to focus on their specific application – and not on the technology.
In interaction with SICK Appspace and SICK’s know-how in the field of sensor applications, the deep learning-based pallet identification system was developed – a complete solution with maximum customer benefit from a single source..
Market relevance
Customers from various industries lose a lot of money every year because deposit pallets are incorrectly assigned in incoming goods. The SICK system helps them avoid losses, save resources, and significantly increase their process quality. A previously resource-intensive and error-prone manual process can be automated in this way. Higher reliability, increased efficiency, and transparency are just some of the benefits ensured by pallet identification from SICK.
With this innovative solution, customers from all industries and across all sectors can optimize their pallet handling and relieve the strain on their incoming goods resources.
Moreover, the market may be significantly larger from the user’s point of view: The technologies used not only lend themselves to pallet type identification applications but can also offer significant benefits in many other areas.
Main customer benefits
The advantages of deep learning-based pallet identification PACS are evident in the creation, deployment and maintenance of the solution.
Time and complexity can be saved in the creation of the solution compared to the use of traditional image processing. By using trained neural networks, it is possible to identify the branding of pallet types with a high success rate, even if the quality is subject to large deviations. These deviations are taken into account in the training process and are learned, thus increasing the robustness of the evaluation.
The use of the solution not only enables an increase in efficiency and throughput, but also an increase in process quality and traceability. Employees are relieved and can concentrate on processes with higher added value.
The space-saving design means that the system can also be integrated in tight assembly positions. The use of standard sensor technology makes maintenance and servicing of the system very cost-effective.
Summary
One of SICK’s customers was faced with the problem that its employees in goods receipt had to manually determine whether incoming pallets belonged to a pallet pool and were pawned accordingly. Until now, this was an additional task in goods receiving that was resource-intensive and error-prone.
With deep learning-based pallet identification PACS (Pallet Classification System) from SICK, customers are given the opportunity to automate a previously laborious and manual process based on a modular kit of hardware and software.
The building block is based on SICK Appspace and dStudio. Appspace is an innovative approach for the realization of powerful apps on SICK sensors, dStudio is a web-based tool for the classification of images based on artificial neural networks, which can be used on SICK sensors. The construction kit can also be used for other tasks in the field of image processing.
IFOY TEST REPORT
Is it a Euro, a Chep, a UIC or perhaps a completely different pallet? Wrong deposit pallets, which are wrongly regarded as “real” deposit pallets at goods receipt and are accepted without complaint, cost companies thousands of euros every year. The PACS application from Sick now wants to put an end to this by enabling the automatic deposit of different pallet types.
PACS – this acronym stands for “Pallet Classification System”, i.e. a recognition system that uses images to identify the type of pallet. Four pictures of the pallet feet are necessary for this, the fifth picture is taken from above and shows what is on the pallet. The whole thing is usually integrated into the stationary conveyor system directly in the goods receiving area; for the test setup in Dortmund, a Sick employee still had to manually feed the incoming pallet onto the camera technology for demonstration purposes. The aim of the event is to provide companies from industry and trade with full transparency in goods receiving and consequently in the flow of goods. Because wrong deposit pallets cost companies a lot of money.
Until now, employees had to manually determine whether a pallet was “good” or “bad” when it entered the factory gate – an additional task that was error-prone and resource-intensive. With the PACS deep-learning pallet identification system, customers are given the opportunity to automate this process on the basis of a modular system of hardware and software. The financial aspect is the direct benefit of this classification system. But there is also an indirect effect, in fact several, that make this solution so interesting. Because incorrect pallets in the system also lead to damage and expensive downtimes of the conveyor system more often than average. Another, even more valuable aspect is more transparent processes by connecting the goods to the load carrier. And finally, no specialised personnel is required to operate the PACS system.
How does PACS work? The hardware of the system consists of one or more cameras with which the images for the system are taken, a light barrier arrangement for triggering and a controller for processing the recorded data and for executing the trained neural network. Even without in-depth knowledge of programming or machine learning, the software tools AICK Appspace and dStudio enable image recording, training, classification and execution of the trained network. Optionally, even further Sick sensors can be integrated, which can take over additional tasks. No programming knowledge is required because the system learns from concrete examples. In this way, Sick was able to make pallet identification comparatively simple for its customers.
Because Sick uses trained neural networks for its solution, the brandings of the individual pallet types can be recognised with a high success rate – even if their quality is subject to large deviations. The training process takes these deviations into account, learns them and thus increases the robust evaluation. Due to its space-saving design, the PACS system can also be integrated in narrow assembly positions. Because Sick uses standard sensor technology for its solution, the system is very cost-effective to maintain and service.
IFOY Test Verdict
With an estimated 500 million Euro pallets in circulation, not to mention the other types, the savings potential through PACS is in the tens of millions. But also companies that regularly use, for example, mesh pallets or small load carriers in their intralogistics can use PACS. The application range of the very reliable pallet classification system covers a broad spectrum: retail, freight forwarding, automotive, mechanical engineering and many other industries.
IFOY INNOVATION CHECK
Market relevance: Given the enormous quantity of goods of all kinds handled on different types of pallets, the Pallet Classification System from Sick is expected to have a very high market relevance. Optimising pallet handling can be beneficial in many areas for a large number of customers. The savings potential in terms of the automation of a former manual work process and the controlled labelling lead us to expect a high level of interest.
Customer benefit: Users ultimately benefit from the cost savings. Pallets do not have to be recorded manually when goods are received and can be automatically recognised and classified with the system. Deposit pallets can be assigned with high accuracy, which brings further cost-saving potential for companies. The expandability of the artificial neural network (ANN)-based system with regard to defect detection should also be emphasised. Faulty pallets can be detected in time, which allows one to take action before subsequent work processes and avoid possible downtimes.
Novelty: Image processing by means of ANNs is not new in itself, but here in practical application it represents an innovative solution for pallet classification. The system is well equipped for the future and can be extended for new pallet types up to the digital pallet (e.g. iPAL). Additional characteristic data can be integrated into the registration and passed on for the customer-specific systems.
Functionality / type of implementation: The system appeared very well implemented and can be flexibly positioned on the conveyor system. The image capture is limited to the lateral capture of the pallet. The detection accuracy depends on the trained ANN in the system, which can be retrained at any time, e.g. for new pallet types.
Verdict: The Pallet Classification System from SICK is undoubtedly a nominee for the IFOY AWARD that promises high savings potential for many areas.
market relevance ++
customer benefit ++
novelty +
functionality / type of implementation +
[++ very good / + good / Ø balanced / – less / – – not available]
For an overview of all the finalists, visit www.ifoy.org
CLICK HERE to find out more about SICK.