Vision-guided robotics & AI: a guide for the non-technical
19th July 2021
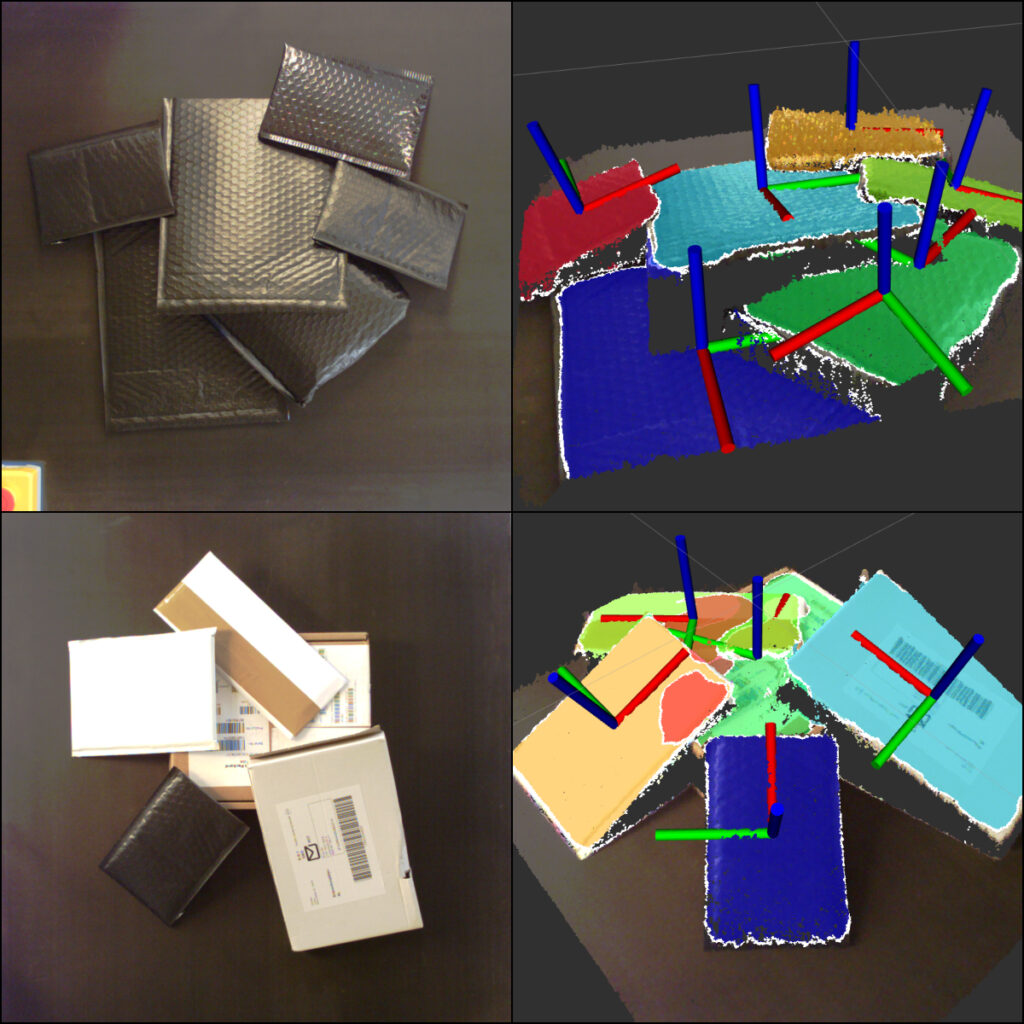
The automation industry is experiencing an explosion of growth and technology capability. To explain this complex technology, we use terms such as “artificial intelligence” to convey the idea that solutions are more capable and advanced than ever before. If you are an investor, business leader, or technology user who is keen to understand the technologies you are investing in, this article will help you gain a well-rounded view on vision-guided robotics and enable you to make informed decisions.
Types of vision systems used in warehousing and distribution environments
There are three primary applications of vision systems used in warehousing and distribution environments:
Inspection and mapping
Vision systems for inspection are used in a variety of industrial robot applications, providing outputs of “pass/fail”, “present/not present”, or a measurement value. The result dictates the next step in a process.
Mapping systems, on the other hand, are less frequently used but are similar to inspection systems in that vision maps do not directly translate into machine action. Both systems can be very sophisticated, but they do not require deep learning or artificial intelligence.
Pick-and-place without deep-learning
Pick-and-place vision systems with limited variables are deployed on most robotic cells installed today. The cameras direct the robot’s motion through closed-loop feedback, enabling the robots to operate very quickly and accurately, within their prescribed parameters. These systems do not have a “learning loop” but are instead pre-programmed for a fixed set of objects and instructions. While these systems are “smart”, they do not add intelligence or learning over time.
Pick-and-place with deep-learning
The most sophisticated vision systems employ “deep learning”, also referred to as “artificial intelligence”. However, many non-learning systems are marketed as if they have intelligent (learning) capability, leading to confusion. The deep-learning algorithms, subset of artificial intelligence, learn features that are invariant of the objects, in order to generalise over a wide spectrum of objects.
For example, through such algorithms, robots can recognise the edge of an object no matter the exposure of the camera or the lighting conditions. Something as simple as a change in lighting could affect the results and that is the reason behind deep-learning systems not relying on a single variable like colour.
It is crucial to note that all three types of vision systems include three main elements: an input (camera), a processor (computer and program), and an output (robot). They may use similar cameras and robots, but the difference lies in the program.
Basic building blocks for deep-learning systems
Vision-guided robots using deep-learning algorithms for industrial applications recognise various types of packaging, location, and other variables (e.g. overlapping items) and act based on those variables. Compared to self-driving cars, some variables for industrial robots are not as complex, but the underlying approach to learning and responding quickly is the same.
There are three co-dependent requirements for deep-learning solutions:
- Computer processing power
- High-quality and varied data
- Deep-learning algorithms
Fizyr has optimised these three elements required for deep-learning systems.
Deep-learning vision systems for vision-guided industrial robots
Commercial applications using robots to pick, place, palletise, or de-palletise in a warehouse environment require three basic building blocks: cameras, software, and robots. The cameras and robots are the eyes and arms; the software is the brain. All three components must work together to optimise system performance.
Camera technology enables the flow of high-quality data. Cameras and post-image processing provide a stream of data ready for the deep-learning algorithm to evaluate. Some cameras are better suited for an application, but that itself is not what makes a vision-guided robot capable of deep learning. The camera supplies data but does not translate data into actionable commands.
This is where the role of software comes in, which is the deep-learning algorithm – data in from cameras, process, results out to robots.
The robot and end-effector (a.k.a. gripper) also play a critical role in system performance. They must provide the level of reach, grip-strength, dexterity, and speed required for the application. The robot and end-effector respond to commands from the deep-learning algorithm.
Conclusion
To summarise, there are three points to remember about artificial intelligence and vision-guided robotic systems:
- Deep-learning algorithms classify data in multiple categories
- Deep-learning algorithms require both high-quality and varied data
- Algorithms become more powerful over time
Latest developments in camera technology and computer processing power serve as building blocks to advanced deep-learning software that improves robot performance. The future has arrived!